In this Research Highlight, Dr Paul Sapin, a Research Associate in the Clean Energy Processes Laboratory at Imperial College London’s Department of Chemical Engineering, explains how Project 2 of the Integrated Development of Low-carbon Energy Systems (IDLES) programme is building a collection of comprehensive thermodynamic and costing models for energy technologies to determine the role they might play in an integrated future energy system.

The transition to a low-carbon sustainable energy system is a challenge being tackled by large numbers of engineers, scientists, economists and policy makers globally. Reshaping the energy system to be cost-effective and environmentally efficient calls for a holistic approach, as identified under the Integrated Development of Low-carbon Energy Systems (IDLES) programme, which is tasked with identifying the ‘best’ pathway to decarbonise the energy sector.
A key question within the energy sector – not just in the future, but now – is which energy technologies could, or should, be playing a leading role? This far-reaching question affects society all the way from national governments (e.g., in considering whether to upgrade the existing gas network to utilise hydrogen for heating homes) through to individual energy users (e.g., in considering whether to purchase a heat pump for their home). And we are not just talking about established technologies commercially available today. There are technologies that, perhaps, are not yet financially viable, as well as those further back in the research pipeline that may one day have a role to play.
Where to find a reliable portfolio of energy technologies?
The landscape of available solutions is growing rapidly. However, the absence of an appropriate database to catalogue these solutions – one which captures operational and performance characteristics at an appropriate level of accuracy and reliability – means that it is not possible to properly examine their role in an integrated future energy system.
In Project 2, our work is therefore to characterise energy generation, conversion and storage technologies so that their inclusion in a whole-system model can reveal their value (e.g., in providing flexibility and adaptability) for the energy system. This research activity, led by Prof. Christos Markides, head of the Clean Energy Processes (CEP) Laboratory, involves Andreas Olympios, Matthias Mersch, Antonio M. Pantaleo and myself, along with other members of the CEP Laboratory.
Technology development is a result of many decisions that affect material and component selection and design, arising from trade-offs between performance, cost and scale economies, usage characteristics, degradation, reliability, lifetime and learning curves. For example, a heat pump manufacturer will develop a product optimised from the manufacturer’s perspective for a particular application in a particular market segment. This product, in turn, comprises components and bespoke control systems, selected specifically for this application.
Our ambition is not only to assess the performance and cost of readily-available systems but also to reveal the untapped potential of existing technologies and unlock that of emerging, disruptive solutions. To do so, we are building a collection of comprehensive thermodynamic and costing models for energy technologies, considering them all the way from the molecular level up to their integration into the whole energy system. This holistic approach allows us to capture the underlying physics and to account for the mechanisms that affect the performance of said technologies. We are compiling them into an open-source technology model library, which will soon be made available on Github.
Despite the clear utility and value of whole-energy systems approaches, many have been limited to date by the use of simplified cost and performance assessment methods, typically using single, fixed values for the efficiency and specific costs of energy conversion or storage technologies. Our group has found that these simplifications can lead to large inaccuracies and result in different decarbonisation pathways being chosen by the energy system model.
Providing rich, reliable and accurate technology models to the central whole-system modellers in IDLES is thus paramount to effectively support the evolution towards a clean, sustainable energy future. There is a limit though. The more details you encapsulate, the more computationally demanding your models become, hence making the whole optimisation problem near impossible to solve. A trade-off must then be found between the granularity of the technology models (i.e., the level of details in the description of the system inner workings) and the associated computational resources.
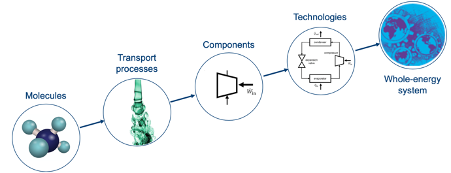
So, what are the options?
To answer that question, let us consider the example of a well-known, strong candidate technology for the provision of low-carbon heating and cooling: the heat pump. Mechanical heat pumps, also known as vapour-compression heat pumps (VCHPs), are a great illustration of the complexity of thermo-mechanical systems modelling, as they are both a highly mature (all refrigeration systems, including your home fridge, are basically heat pumping systems) and constantly-evolving technology.
A steak and stilton pie served piping hot from the oven cools down while sitting on your plate. In other words, heat is spontaneously transferred from the warmer body to the colder one. A mechanical heat pump is basically an electrically-driven thermodynamic device that moves thermal energy in the opposite direction.
The working principle of the VCHP was first proposed in the 1800s, while their rapidly-growing commercialisation started in the 1950s. Mechanical heat pumps use work to extract heat from a cold source (typically the outside air or the ground) and upgrade it to a higher temperature so that it can be used, for example to heat up your home. They consist principally of four components – namely, an evaporator, a condenser, a compressor and an expansion valve. A working fluid (commonly referred to as the refrigerant) is circulated by the compressor (typically powered by an electric motor), condensed at high pressure and temperature, thus releasing heat to the heat sink (the house in the case of a domestic heat pump), and finally evaporated at lower pressure and temperature, thus sucking in heat from the cold heat source.
The technical performance of a heat pump is measured by the so-called coefficient of performance (COP), defined as the ratio of the provided heating power to the electricity consumed. As a rule of thumb, it is often assumed that air-source heat pumps exhibit a COP of 3, while ground-source heat pumps typically exhibit COPs around 4. In other words, an air-source heat pump sucking heat from the outdoor air is expected to consume 1 kW of electricity to provide 3 kW of heat, while ground-source heat pumps would provide 4 kW of heat with the same electrical consumption. These figures, though realistic, are rough estimates of the performance that a real heat-pumping system would achieve, unable to capture the performance variations with climatic conditions for example. Plugging such uncertain indicators into a whole-energy system optimisation framework may lead to unfeasible heat-decarbonisation pathways or to a failure to identify the most promising strategies.
At a slightly higher level are technology-agnostic models. This is also a relatively simple and quick approach. Technology-agnostic methods aim at providing realistic estimates of a given technology performance solely from the external operating conditions, e.g., solely from the knowledge of the ambient air temperature and desired heating temperature for an air-source domestic heat pump. True to their name, technology-agnostic models do not require anything about the system inner workings to be specified to determine the potential of a given solution, which makes them highly attractive. In particular, they are able to provide realistic performance estimates in time-varying operating conditions or to compare the performance in various locations and climates. On the downside, these models do not capture the effects of the system size and design and hence do not provide information for refined costing.
The next level up is to carry out a data-driven techno-economic assessment, which is more practical by nature. As their name suggests, data-driven models are built using actual, lab- or field-scale data for the technology being studied, typically from a market-based analysis using manufacturer data. The main advantage of this approach is that it provides a comprehensive link between cost and performance. In addition, uncertainty bounds are derived for all key performance indicators, which is of great value. As argued by Contino et al. [1], once an optimal pathway is identified using a whole-energy model, the what if questions remain open: typically, what if the price of heat pumps or cost of electricity were uncertain? Different educated guesses on the latter could lead to radically different so-called optimal development pathways. The quantification of the uncertainty on the model parameters and their integration into the whole-system analysis is the only way to address this issue. Optimisation under uncertainty is key to identifying not just the best route but the best route that we know to be feasible with high confidence.
Data-driven techniques are thus inherently realistic and robust. However, they can be highly uncertain as well, especially for mature technologies. Let us illustrate this point by means of the heat-pump example. As a highly mature technology, a very wide range of heat-pump systems have been custom designed by manufacturers for various applications and different market segments: from cheap, low-performance designs to expensive, high-performance ones. Classifying existing systems into low- to high-spec categories is a risky exercise as most manufacturers reveal very little of their design choices, material and components selection, or control algorithms. This leaves us with scattered data, as illustrated by Olympios et al. [2] who report uncertainties on the cost of air-source heat pumps larger than 50%, thus providing us with an unclear link between the cost of a system and its expected performance.
Another, inherent limit of data-driven methods is that they are restricted to existing systems, as field-scale or manufacturer data are obviously not available for novel technologies. Typically, what about tomorrow’s heat pump? Will it use similar materials, fluids, components, controls? Are today’s observed cost-performance relationships representative of tomorrow’s situation? This is very unlikely. For example, the Kigali amendment to the Montreal protocol, agreed in 2016, aims at phasing down and, ultimately, phasing out the use of high-GWP (global warming potential) working fluids in refrigeration and heat-pump systems, currently still widely used. Tomorrow’s heat pumps will thus use replacement flammable (such as hydrocarbons), high-pressure (e.g. carbon dioxide) or toxic (ammonia) working fluids, requiring different designs, materials and controls, leading to different cost-performance relationships.
Developing comprehensive cost and performance-prediction methods is thus required to properly assess and compare the potential of both available and under-development technologies. Unlike technology-agnostic and data-driven assessment techniques, first-law comprehensive models are based on a detailed description of the technology components (dimensions, types, materials) and available controls. The impact of all loss mechanisms (e.g., heat transfer, friction, pressure losses, mass leakage) are carefully quantified through the resolution of mass- and energy-conservation equations. Along with advanced optimisation algorithms, these deterministic models allow us to investigate the techno-economic performance of low- to high-spec designs and thus capture the complexity of thermo-mechanical technologies with high confidence.
These computationally-expensive methods are key to: (i) provide a fair comparison between novel and mature technologies; (ii) account for application-specific constraints (e.g., heat pump defrost in cold climates, noise limitations); (iii) assess and unlock potential of disruptive innovating technologies; (iv) provide guidance to manufacturers; and (v) anticipate on future regulations for the technology (e.g., phasing out of high-GWP refrigerants).
How do we know our approach works?
Alongside the computational work, we also conduct experimental trials on custom testing facilities designed and assembled within the lab. At the moment, for example, we are investigating the performance of reciprocating-piston devices using a dedicated test bench featuring advanced electromagnetic valve actuation, a schematic of which is shown below. Time-resolved pressure, temperature, torque, power and flow rate measurements allow us to determine the performance of the camless reversible compressor/expander in part-load and off-design operating conditions. The high-fidelity test data gathered will be used not only to verify the accuracy of, and inform, our models, but also to develop bespoke control algorithms for various applications (e.g., advanced heat pumps, large-scale energy-storage technologies).

Which modelling option to choose then?
In a nutshell, all the different cost and performance prediction methods, even the simplest, less detailed ones, bring advantages along with drawbacks. None should be eliminated from scratch. Defining the right, adequate modelling granularity of a given technology for a specific optimisation problem is highly application specific. This is why we endeavour to provide comprehensive first-law and costing models together with data-driven and technology-agnostic assessment techniques for each technology in the library that we are compiling. This multi-fidelity technology model portfolio will soon be made available open-source on the Github platform.
Works Cited
[1] F. Contino, S. Moret, G. Limpens and H. Jeanmart, “Whole-energy system models: The advisors for the energy transition,” Progress in Energy and Combustion Science, vol. 81, 2020.
[2] A. V. Olympios, A. M. Pantaleo, P. Sapin and C. N.Markides, “On the value of combined heat and power (CHP) systems and heat pumps in centralised and distributed heating systems: Lessons from multi-fidelity modelling approaches,” Applied Energy, vol. 274, p. 115261, 2020.
Biography

Dr Paul Sapin is a Research Associate in the Clean Energy Processes (CEP) Laboratory, Department of Chemical Engineering. Paul works on Project 2 of the IDLES Programme, investigating the capabilities, performance and cost of energy technologies from a whole system perspective. As leader of the Energy Division of the CEP Laboratory, he undertakes research on a wide range of thermo-mechanical energy technologies, through the use of joint experimental and numerical approaches. Applications of his research include energy storage (e.g., compressed- or liquid-air electricity storage and Carnot batteries), waste-heat recovery (e.g., using organic Rankine cycle and Joule/Brayton cycle engines) and decarbonisation of heating/cooling provision (e.g., with mechanically- and thermally-driven heat pumps).